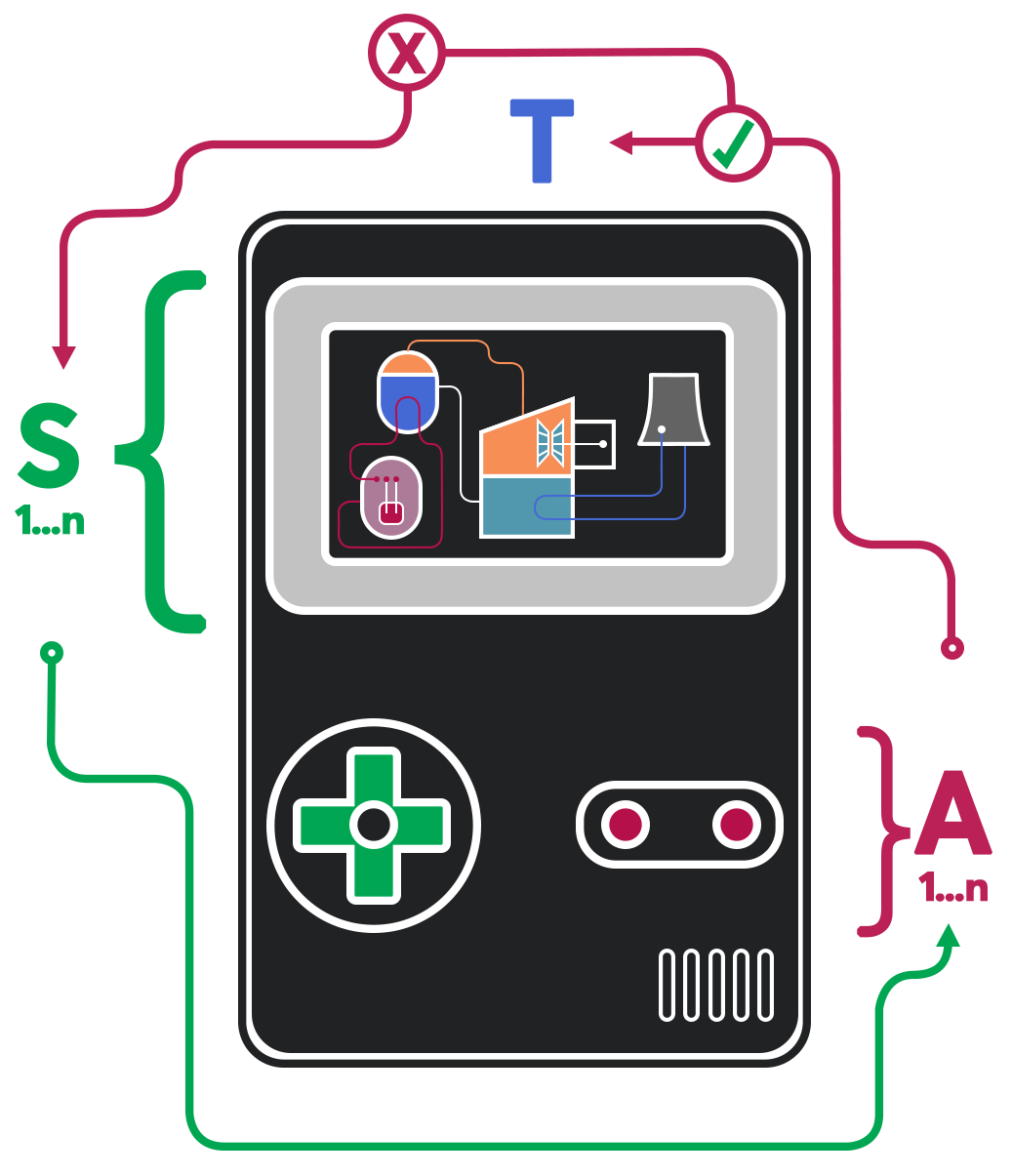
Space-Time dependencies
Build an abstract representation of your process through collaboration & smart recommendations.
SQUIDBOT.io is a deep learning hub for manufacturers where engineers build and train industrial agents in minutes. The Space-Time module will guide you easily in building how your agent perceives information and proposes decisions accordingly.
Guide your agent through Pathemata Mathemata
There will be no better approach than teaching agents than Pathemata Mathemata: learning through pain. In other words, it is much better to teach agents on every piece of information, including bad information.
User-friendly space-time integration module
The Space-Time module provides engineers with user-friendly and intuitive commands that can make spatial & temporal parameter setting simpler.
-
User-friendly guide on Space-Time dependencies
Users are guided in simple steps to understand the logic behind the agent learning process: interaction with environments & decision spaces.
-
Exploration/Exploitation Tradeoff
Should industrial agents explore more or just get along with exploited targets? It is easier to tune this parameter from now on.
-
How to design state, action & target vectors
Your agents targets are just the best states resulting from great actions.
Make your production more convex
Designing the right space-time environment makes your agents more convex to volatility
-
Risk mitigation
Convexity allows your agents to be more prepared for eventual volatility
-
Reality is probability
The better your space-time medium, the closer you get to reality